How NLP use cases can differentiate your business and customer experience
Summary
Learn how three companies are utilizing NLP to improve customer, employee and patient experiences.
Read time: 5 minutes
In today’s competitive business environment, everyone is searching for differentiation to show customers they’re the best at what they do. Using the right technology in the right way can separate your business from the rest of the pack.
In our last innovation blog on natural language processing (NLP), we looked at the landscape of AI investments and growth as well as the types of data and use cases that are ripe for improvement. In this second article on NLP uses, let’s look at the process and see how several customers innovated their businesses.
Using NLP and automating the quality assurance checkpoint reduced operation costs, increased quality service, improved data accuracy, and saved 44 hours per month by eliminating manual labor and employees managing the antiquated text-mining tool.
Six focus areas of NLP
In document digital transformation, there are often a series of automation steps that utilize AI and natural language processing (NLP). After documents, images, or files are digitized, they go through pre-processing steps (optical character recognition or OCR, speech-to-text, handwriting recognition and/or other conversions) so the computer can then transform information into actionable data.
The transformation of information into data is the critical juncture where organizations can apply NLP. NLP pre-processes data and develops algorithms using different types of AI tools — machine learning, deep learning, and neural networks — to help businesses quickly extract, classify, and analyze massive amounts of data.
Here are six areas to consider where NLP can add value in document digital transformation workflows, depending on the use case and requirements:
Classification: Classifies or indexes documents into predefined categories according to the intent. Using NLP for classification eliminates manual data entry and increases productivity, consistency, and accuracy.
Regression: This process calculates scores from the intent of the document. The benefit is quick prioritization for faster responses.
Extraction: NLP is used to pull data out of unstructured documents and identify portions of a document that meet specific criteria. This technology accelerates processing, increases accuracy, and reduces costs.
Sequence Matching: The application can look at multiple documents with the same meaning and reduces time spent finding and sorting documents resulting in efficiency, productivity, and cost-savings.
Clustering: NLP can be used to visually show and group multiple documents with similar intents. A visual display of data can lead to faster decision-making and insights as well as assist with identifying knowledge gaps.
Sequence Generation: With sequence generation, the application takes an inquiry (a sentence) and generates a reply. Smart tools, like chats or bots, can enhance customer experiences with fast replies and information.
In the above diagram, any combination of the NLP areas can be incorporated into the “Analyze & Generate” automated workflow step. Once the information is transformed into structured data, the platform can deliver or export it to any other application, repository, or workflow.
NLP use case #1: Customer call center workflow
With revenues over $7B, a large food manufacturing company’s call center, which handled over 60,000 calls per month, was overwhelmed and needed to quickly prioritize complaints. It was difficult with their legacy, inaccurate text-mining tool and required significant manual work, especially for the quality assurance department.
Using Ricoh Integrated Solution for a web application, the company automated 98% of its call center workflow. Now, when inquiries come into the call center, a needs analysis transforms the data using classification and regression modules. The computer classifies the call into four categories: compliment, request, advice, or complaint. There are also classifications for food safety (8 types that the computer can select), manufacturing and distribution (5 types or options), and needs categories (4 types) that the application identifies and routes to the right department. The regression analysis scores and prioritizes the issues depending on the classification types.
Automating the quality assurance checkpoint reduced operation costs, increased quality service, improved data accuracy, and saved 44 hours per month by eliminating manual labor and employees managing the antiquated text-mining tool.
NLP use case #2: Knowledge gap analysis
For a $2B device manufacturing company, the number of inbound calls was increasing annually to over 214,000 per year. Management wanted faster response times, a reduction in call volume, higher accuracy logs, and better customer support.
With the new Ricoh Integrated Solution in place with NLP, they implemented a clustering module. The inquiries and service requests would come into the call center and be entered into a data log, which was then clustered (grouped) in a map. The employees could now visually see the balance of customer inquiries and knowledge articles being used (refer to image). The clusters also alerted employees about unsupported inquiries, which were now visually easier to find.
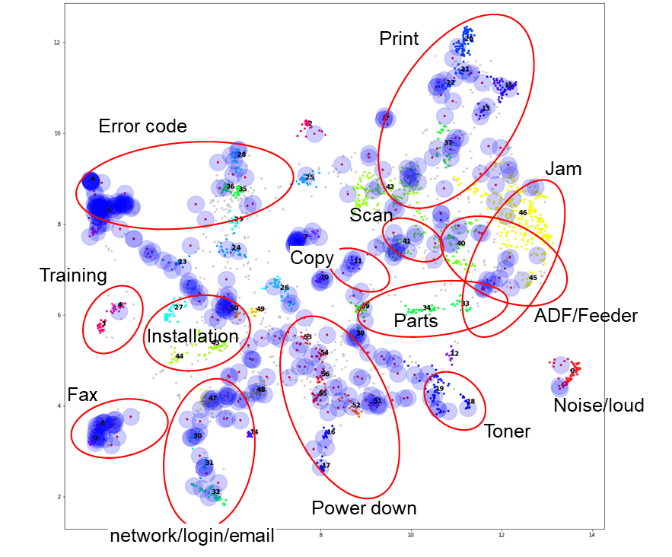
The company could now proactively create new knowledge articles to avoid customer calls and improve service quality based on the clustering maps. Additionally, the company used this information to create a robust frequently asked questions (FAQs) guide to make it even easier for customers to find solutions on their own, reducing knowledge gaps. The call center employees were able to reduce their weighty workload, relieving stress and overtime.
NLP use case #3: Proactive patient care
A $332M nursing care facility wanted to improve the caregiver’s medical analysis to ensure the best care was given to every patient. There was increased pressure on staff for reporting due to several instances of patients’ conditions worsening without understanding the cause. The healthcare facility wanted to create a system of evaluating both the caregiver and the patient, with the ability to send preventative alerts to staff.
Using a document digital transformation (DDX) application with AI and NLP to sift through the daily reports, a voice recording could be added to the patient’s medical record. The AI would automatically list potential issues and their severity, enabling staff to prioritize and determine the best outcome. The DDX application could identify and suggest, based on the patient’s condition described in the daily report, the care they may need to provide.
NLP technology enabled both the extraction of data from the recording and medical records and the capability to run regression testing and scoring. The extracted data was used to create daily predictive alerts and monthly reports. The regression module scored and prioritized patient care, predicting if they needed to be transported within two weeks. The staff was better equipped to respond to at-risk patients and the quality of care improved. The staff’s service quality was also run through regression analysis to ensure proper care.
Rising above to deliver excellent customer experiences
These success stories describe how enterprises are not only improving the experiences of customers but how the technology enables their employees to be better at their jobs with the right data and a balanced workload. Document digital transformation is one of the easiest and most impactful ways to grow your business. What are your biggest business challenges that NLP can tackle?
Stay tuned for our next innovation topic with five tips to start a co-innovation NLP initiative with Ricoh.
Get started with NLP and co-innovation.
Kazutaka Oba
Recommended for you
Navigating NLP for Growth Opportunities
The first article in our innovation series explores today's NLP and generative AI landscape along with ideas on how NLP opportunities lead to growth.
Growth and manufacturing innovation
Enhance manufacturing innovation while supporting healthy manufacturing plant growth by utilizing digital and advanced manufacturing technologies.
Benefits of AI-driven insurance claims management
Confronted with a customer now demanding instant gratification, insurers are laser-focused on their automating their claims management processes using AI technology.